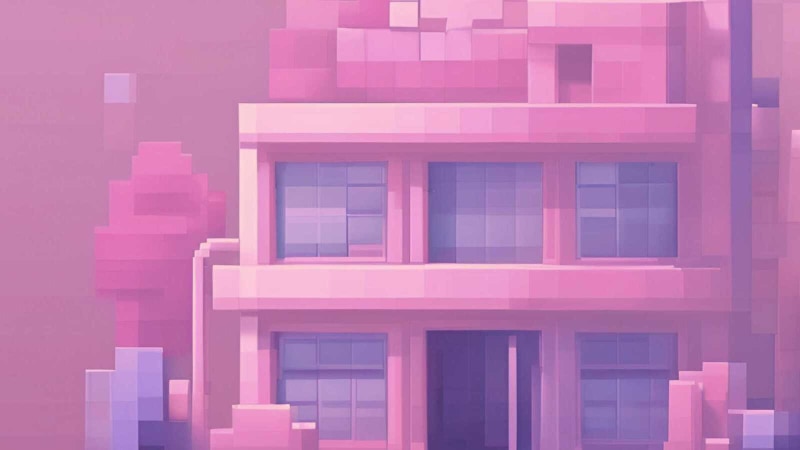
Ethical and Regulatory Challenges of Applying Artificial Intelligence in Healthcare
November 19, 2024
Integrating Artificial Intelligence (AI) in healthcare is a revolutionary shift that offers transformative potential in diagnostics, treatment planning, and patient care. However, its adoption comes with ethical, practical, and legal challenges that demand a strong regulatory framework. Understanding these frameworks is crucial for stakeholders who need to balance innovation with patient safety, privacy, and trust.
Key Regulatory Insights for AI in Healthcare
1. Understanding Existing Regulatory Frameworks
AI in healthcare is often regulated under frameworks designed for Software as a Medical Device (SaMD). While these frameworks provide a foundation, they constantly evolve to address AI’s unique characteristics, like adaptability and machine learning capabilities.
2. Risk-Based Classification
A risk-based classification approach is a common method for regulating AI systems.
- Example: The EU's AI Act categorizes AI systems into risk levels, with high-risk applications, like diagnostic tools, subjected to stricter regulations. Lower-risk AI applications, such as administrative tools, often follow voluntary codes of conduct.
3. Total Product Life Cycle (TPLC) Approach
The U.S. FDA champions the TPLC approach, emphasizing continuous oversight of AI systems throughout their lifecycle.
- Components of TPLC:
- Pre-market assessment
- Post-market surveillance
- Ongoing management of updates and algorithmic changes
4. Transparency and Explainability
A significant regulatory challenge lies in AI's "black box" nature—where decision-making processes can be opaque. Regulators now emphasize the importance of:
- Transparency in how AI systems function
- Explainability of decisions made by AI
- Clear documentation on the data and algorithms driving AI outcomes
5. Data Governance and Privacy
AI in healthcare requires access to sensitive patient data, raising concerns about privacy and security. Regulations such as the EU's General Data Protection Regulation (GDPR) enforce stringent measures, including:
- Explicit patient consent
- Data anonymization
- Secure storage protocols
6. Accountability and Liability
Determining accountability for AI-driven decisions is complex, especially as systems become more autonomous. Clear guidelines are necessary to define the responsibilities of:
- AI developers
- Healthcare providers
- Institutions utilizing AI systems
Recommendations for Navigating AI Regulations
1. Stay Informed
The regulatory landscape for AI in healthcare is dynamic. Regularly monitor updates on laws, standards, and guidelines in your region to remain compliant and competitive.
2. Engage with Regulators
Collaborate with regulatory agencies to:
- Clarify requirements
- Address potential concerns
- Foster a cooperative environment for AI adoption
3. Prioritize Ethical Considerations
Ethical principles should guide AI development and deployment. Focus on ensuring:
- Fairness
- Transparency
- Accountability
4. Implement Robust Data Governance
Compliance with data protection regulations is essential. Establish clear protocols for:
- Data collection and anonymization
- Secure storage
- Controlled data sharing
5. Focus on User Education
Educating healthcare professionals and patients about AI’s capabilities and limitations builds trust and ensures responsible use.
The Path Forward
Successfully integrating AI in healthcare demands a balanced approach that promotes innovation while safeguarding patient safety, privacy, and ethical integrity. A robust regulatory framework, combined with proactive engagement from developers, healthcare providers, and regulatory bodies, is essential to mitigate risks and unlock AI’s transformative potential.
By navigating these regulations effectively, stakeholders can drive meaningful advancements in healthcare while maintaining the trust and well-being of patients. This structured approach ensures compliance and positions organizations as leaders in ethical and innovative AI deployment in healthcare.
AI in Healthcare: An FAQ
1. What is AI and how is it being used in healthcare?
AI, or Artificial Intelligence, is a branch of computer science that involves creating intelligent agents, which are systems that can reason, learn, and act autonomously. In healthcare, AI is being used in a wide range of applications. These applications fall into two main categories:
- Diagnosis support systems: These systems analyze patient data, medical records, and clinical information to assist healthcare professionals in making accurate diagnoses and treatment plans. Examples include AI-powered image analysis tools for early disease detection and systems that analyze patient data to predict disease risk.
- Care assistive systems: These systems help individuals manage their health and wellness, often through the use of wearable technology and digital self-management applications. These systems can monitor patients' vital signs, provide medication reminders, and offer personalized health advice.
2. How is AI transforming drug discovery and treatment?
AI is revolutionizing drug development in several ways:
- Accelerated drug discovery: AI algorithms can analyze vast datasets of chemical and biological information to identify potential drug candidates and predict their efficacy. This significantly reduces the time and costs associated with traditional drug discovery methods.
- Personalized medicine: By analyzing individual patient data, AI can help tailor treatments to specific needs and predict how patients will respond to different medications. This enables more effective and precise therapies.
- Optimized drug delivery: AI-powered micro- and nanosensors can monitor drug concentrations in the body and adjust dosages in real-time, leading to improved treatment outcomes and reduced side effects.
3. What are the ethical considerations surrounding AI in healthcare?
The use of AI in healthcare raises several ethical considerations:
- Privacy and data security: AI systems rely on large amounts of patient data, raising concerns about privacy and the potential for data breaches. Protecting sensitive patient information is crucial.
- Bias and fairness: AI algorithms can inherit biases present in the data they are trained on, potentially leading to disparities in healthcare access and treatment. Ensuring fairness and equity in AI applications is essential.
- Transparency and explainability: The decision-making processes of complex AI systems can be difficult to understand, making it challenging to determine accountability when errors occur. Transparency and explainability are crucial for building trust in AI-based healthcare.
4. How is the doctor-patient relationship impacted by AI?
The integration of AI in healthcare is redefining the doctor-patient dynamic:
- Shifting roles: AI empowers patients to take a more active role in managing their health, leading to a more collaborative relationship with healthcare providers.
- Enhanced communication: AI-powered tools can improve communication by providing patients with personalized information and enabling more efficient interactions with healthcare professionals.
- Potential for dehumanization: Over-reliance on AI could diminish the human element of healthcare, impacting the emotional support and empathy that are essential aspects of the doctor-patient relationship.
5. What are the key regulatory challenges for AI in healthcare?
Regulating AI in healthcare poses unique challenges:
- Evolving technology: The rapid pace of AI advancements makes it difficult to establish regulations that can keep up with the latest developments.
- Determining risk: Classifying the risk associated with different AI applications is complex and requires careful consideration of their intended use and potential impact on patient safety.
- Balancing innovation and safety: Regulations need to promote innovation while ensuring the safety and effectiveness of AI technologies. This balance is essential for realizing the full potential of AI in healthcare.
6. What are the key differences between LLMs and traditional AI in healthcare?
Large Language Models (LLMs), like ChatGPT, are a new type of AI with distinct characteristics:
- Scale and complexity: LLMs are trained on massive datasets and have billions of parameters, making them significantly more complex than traditional AI systems used in healthcare.
- Generative capabilities: LLMs can generate human-quality text, which opens up possibilities for tasks like drafting medical reports, summarizing research papers, and interacting with patients through chatbots.
- Lack of medical specialization: Most current LLMs lack specific training on medical data, which limits their accuracy and reliability for medical applications.
7. What are the specific regulatory considerations for LLMs in healthcare?
LLMs pose new challenges for regulators:
- New regulatory category: The unique characteristics of LLMs may require creating a new regulatory category specifically designed to address their potential risks and benefits in healthcare.
- Addressing data privacy: LLMs' reliance on vast datasets requires stringent regulations to ensure the privacy and security of sensitive patient information.
- Ensuring accuracy and reliability: Regulators need to establish standards for evaluating the accuracy and reliability of LLMs in medical contexts to prevent harm to patients.
8. How can we ensure responsible development and use of AI in healthcare?
To ensure responsible AI in healthcare:
- Develop robust ethical guidelines: Clear ethical guidelines are needed to guide the development and deployment of AI systems that prioritize patient well-being and avoid potential harm.
- Prioritize transparency and explainability: AI developers and healthcare organizations should strive for transparency in their AI systems, making their decision-making processes understandable and accountable.
- Engage patients and healthcare providers: Actively involve patients and healthcare providers in the development and evaluation of AI systems to ensure they meet real-world needs and address ethical concerns.